Application of Data Mining Technology in Financial Risk Analysis
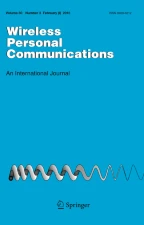
Facing the cruel market competition environment, the enterprise’s demand for risk management is increasing day by day. How to objectively evaluate the financial risks existing in the process of enterprise management and how to timely warn them is the goal that the enterprise management always pursues. Enterprise financial risk analysis and early warning research is influenced by various factors inside and outside the enterprise. The results show that the uncertainty of the technology is very high, and the excellent performance of data mining technology in the study of uncertainty theory links the two closely. In addition, it also proves that the three improved algorithms of association rules greatly improve the efficiency of data mining. Meanwhile, the concept hierarchy tree model of enterprise financial risk and the financial crisis early-warning model of dynamic maintenance of time series are put forward. In conclusion, these algorithms are suitable for the research of enterprise financial risk analysis and crisis warning.
This is a preview of subscription content, log in via an institution to check access.
Access this article
Subscribe and save
Springer+ Basic
€32.70 /Month
- Get 10 units per month
- Download Article/Chapter or eBook
- 1 Unit = 1 Article or 1 Chapter
- Cancel anytime
Buy Now
Price includes VAT (France)
Instant access to the full article PDF.
Rent this article via DeepDyve
Similar content being viewed by others
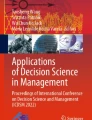
Design of Financial Risk Early Warning System in Enterprise Economic Management Based on Cluster Algorithm
Chapter © 2023
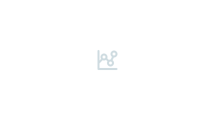
RETRACTED ARTICLE: Research on financial management model based on computer information technology
Article 18 October 2021
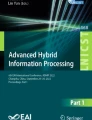
Design of Financial and Economic Monitoring System Based on Big Data Clustering
Chapter © 2023
Explore related subjects
References
- Edwards, M., Rashid, A., & Rayson, P. (2015). A systematic survey of online data mining technology intended for law enforcement. ACM Computing Surveys,48(1), 1–54. ArticleGoogle Scholar
- Lee, J., Lee, J., Kim, G., Park, S., & Jang, D. (2015). Establishment of strategy for management of technology using data mining technique. Journal of Korean Institute of Intelligent Systems,25(2), 126–132. ArticleGoogle Scholar
- Wook, M., Yusof, Z. M., & Nazri, M. Z. A. (2015). The acceptance of educational data mining technology among students in public institutions of higher learning in malaysia. International Journal of Future Computer and Communication,4(2), 112–117. ArticleGoogle Scholar
- Zhang, T. R., Yu, T. B., Zhao, H. F., & Wang, W. S. (2015). Application of data mining technology in fault diagnosis of tunnel boring machine. Dongbei Daxue Xuebao/Journal of Northeastern University, 36(4), 527–531, 541.
- Kao, J. H., Chen, H. I., Lai, F., Hsu, L. M., & Liaw, H. T. (2015). Decision tree approach to predict lung cancer the data mining technology. Lecture Notes in Electrical Engineering,331(2), 273–282. ArticleGoogle Scholar
- Liu, K., Wang, J., & Hong, Y. (2017). Wearing comfort analysis from aspect of numerical garment pressure using 3d virtual-reality and data mining technology. International Journal of Clothing Science and Technology,29(2), 166–179. ArticleGoogle Scholar
- Huang, X., Fan, X., Chen, X., Yang, G., & Gan, M. (2016). Bed permeability state prediction model of sintering process based on data mining technology. ISIJ International,56(12), 2113–2117. ArticleGoogle Scholar
- Margolies, L. R., Pandey, G., Horowitz, E. R., & Mendelson, D. S. (2016). Breast imaging in the era of big data: structured reporting and data mining. American Journal of Roentgenology,206(2), 259–264. ArticleGoogle Scholar
- Carmichael, L., Stalla-Bourdillon, S., & Staab, S. (2016). Data mining and automated discrimination: a mixed legal/technical perspective. IEEE Intelligent Systems,31(6), 51–55. ArticleGoogle Scholar
- Jiang, Y. (2016). Research on e-commerce consumer factors of agricultural products in agritourism based on data mining technology. International Journal of Multimedia & Ubiquitous Engineering,11(11), 297–308. ArticleGoogle Scholar
Acknowledgements
This work was supported by the National Natural Science Foundation of China (Grant No. 71001075), and the Fundamental Research Funds for the Central Universities (Grant Nos. skqy201739, skqy201409 and skzx2017-sb35).
Author information
Authors and Affiliations
- Business School, Sichuan University, Chengdu, China Maozhu Jin, Yanan Wang & Yucheng Zeng
- Maozhu Jin